As early as last fall, before ChatGPT had even launched, experts were already predicting that issues related to the copyrighted data that trained generative AI models would unleash a wave of litigation that, like other big technological changes that changed how the commercial world worked — such as video recording and Web 2.0 — could one day come before a certain group of nine justices. “Ultimately, I believe this is going to go to the Supreme Court,” Bradford Newman, who leads the machine learning and AI practice of global law firm Baker McKenzie, told VentureBeat last October — and recently confirmed that his opinion is unchanged. Edward Klaris, a managing partner at Klaris Law, a New York City- based firm dedicated to media, entertainment, tech and the arts, also maintains that a generative AI case could “absolutely” be taken up by the Supreme Court. “The interests are clearly important — we’re going to get cases that come down on various sides of this argument,” he recently told VentureBeat. >>Follow VentureBeat’s ongoing generative AI coverageThe question is: How did we get here? How did the trillions of data points at the core of generative AI become a toxin of sorts that, depending on your point of view and the decision of the highest judicial authority, could potentially hobble an industry destined for incredible innovation, or poison the well of human creativity and consent? The explosion of generative AI over the past year has become an “‘oh, shit!” moment when it comes to dealing with the data that trained large language and diffusion models, including mass amounts of copyrighted content gathered without consent, Dr. Alex Hanna, director of research at the Distributed AI Research Institute (DAIR), told VentureBeat in a recent interview. The question of how AI technologies could affect copyright and intellectual property has been a known, but not terribly urgent, problem legal scholars and some AI researchers have wrestled with over the past decade. But what had been “an open question,” explained Hanna, who studies data used to train AI and ML models, has suddenly become a far more pressing issue — to put it mildly — for generative AI. Now that generative AI tools based on large language models (LLMs) are available to consumers and businesses, the fact that they are trained on a massive corpora of text and images, mostly scraped from the internet, and can generate new, similar content, has brought about a sudden increased scrutiny of their data sources A growing alarm among artists, authors, and other creative professionals concerned about the use of their copyrighted works in AI training datasets has already led to a spate of generative AI-focused lawsuits filed over the past six months. From the first class-action copyright infringement lawsuit around AI art filed against Stability AI, Midjourney and DeviantArt in January, to comedian Sarah Silverman’s recent lawsuit against OpenAI and Meta filed in July, more copyright holders are increasingly pushing back against data scraping practices in the name of training AI. In response, Big Tech companies like OpenAI have been lawyering up for the long haul. Last week, in fact, OpenAI filed a motion to dismiss two class-action lawsuits from book authors—including Sarah Silverman—who earlier this summer alleged that ChatGPT was illegally trained on pirated copies of their books. The company asked a US district court in California to throw out all but one claim alleging direct copyright infringement, which OpenAI hopes to defeat at “a later stage of the case.” According to OpenAI, even if the authors’ books were a “tiny part” of ChatGPT’s massive data set, “the use of copyrighted materials by innovators in transformative ways does not violate copyright.” The wave of lawsuits, as well as pushback from enterprise companies — that don’t want legal blowback for using generative AI, especially for consumer-facing applications — has also been a wake-up call for AI researchers and entrepreneurs. This cohort has not witnessed such significant legal pushback before — at least not when it comes to copyright (there have been previous AI-related lawsuits related to privacy and bias). Of course, data has always been the oil driving artificial intelligence to greater heights. There is no AI without data. But the typical AI researcher, Hanna explained, is likely far more interested in exploring the boundaries of science with data than digging into laws governing the use of that data. “People don’t get into AI to deal with copyright law,” she said. “Computer scientists aren’t trained in data collection, and they surely are not trained on copyright issues. This is certainly not part of computer vision, or machine learning, or AI pedagogy.” Naveen Rao, VP of generative AI at Databricks and co-founder of MosaicML, pointed out that researchers are usually just thinking about making progress. “If you’re a pure researcher, you’re not really thinking about the business side of it,” he said. If anything, some AI researchers creating datasets for use in machine learning models have been motivated by an effort to democratize access to the types of closed, black box datasets companies like OpenAI were already using. For example, Wired reported that the dataset at the heart of the Sarah Silverman case, Books3, which has been used to create Meta’s Llama, as well as other AI models, started as a “passion project” by AI researcher Shawn Presser. He saw it as aligned with the open source movement, as a way to allow smaller companies and researchers to compete against the big players. Yet, Presser was aware there would be backlash: “We almost didn’t release the data sets at all because of copyright concerns,” he told Wired. But whether AI researchers creating and using datasets for model training thought about it or not, there is no doubt that the data underpinning generative AI — which can arguably be described as its secret sauce — includes vast amounts of copyrighted material, from books and Reddit posts to YouTube videos, newspaper articles and photos. However, copyright critics and some legal experts insist this falls under what is known in legal parlance as “fair use” of the data — that is, U.S. copyright law “permits limited use of copyrighted material without having to first acquire permission from the copyright holder.” At testimony before the U.S. Senate at a hearing on AI and intellectual property related to AI and copyright on July 12, Matthew Sag, a professor of law in AI, machine learning and data science at Emory University School of Law, said that “if an LLM is trained properly and operated with appropriate safeguards, its outputs will not resemble its inputs in a way that would trigger copyright liability. Training such an LLM on copyrighted works would thus be justified under the fair use doctrine.” While some might see that as an unrealistic expectation, it would be good news for copyright critics like AI pioneer Andrew Ng, former co-founder and head of Google Brain, who make no bones about the fact that they know the latest advances in machine learning have depended on free access to large quantities of data, much of it scraped from the open internet. In an issue of his DeepLearning.ai newsletter, The Batch, titled “It’s Time to Update Copyright for Generative AI, a lack of access to massive popular datasets such as Common Crawl, The Pile, and LAION would put the brakes on progress or at least radically alter the economics of current research. “This would degrade AI’s current and future benefits in areas such as art, education, drug development, and manufacturing, to name a few,” he said. But other legal minds, and a rising chorus of creators, see an equally persuasive counterargument — that copyright issues around generative AI are qualitatively different from previous high-court cases related to digital technologies and copyright, most notably Authors Guild, Inc. v. Google, Inc. In that federal lawsuit, authors and publishers argued that Google’s project to digitize and display excerpts from books infringed upon their copyrights. Google won the case in 2015 by claiming its actions fell under “fair use” because it provided valuable resources for researchers, scholars, and the public, while also enhancing the discoverability of books. However, the concept of “fair use” is based on a four-factor test — four measures that judges consider when evaluating whether a work is “transformative” or simply a copy: the purpose and character of the work, the nature of the work, the amount taken from the original work, and the effect of the new work on a potential market. That fourth factor is the key to how generative AI really differs, say experts, because it aims to assess whether the use of the copyrighted material has the potential to negatively impact the commercial value of the original work or impede opportunities for the copyright holder to exploit their work in the market — which is exactly what artists, authors, journalists and other creative professionals claim. “The Handmaid’s Tale” author Margaret Atwood, who discovered that 33 of her books were part of the Books3 dataset, explained this concern bluntly in a recent Atlantic essay: “Once fully trained, the bot may be given a command—’Write a Margaret Atwood novel’—and the thing will glurp forth 50,000 words, like soft ice cream spiraling out of its dispenser, that will be indistinguishable from something I might grind out. (But minus the typos.) I myself can then be dispensed with—murdered by my replica, as it were—because, to quote a vulgar saying of my youth, who needs the cow when the milk’s free?” Two decades ago, no one in the AI community thought much about the copyright issues of datasets, because they were far smaller and more controlled, said Hanna. In AI for computer vision, for example, images were typically not gathered on the web, because photo-sharing sites like Flickr (which wasn’t launched until 2004) did not exist. “Collections of images tended to be smaller and were either taken in from under certain transit controlled conditions, by researchers themselves,” she said. That was true for text datasets used for natural language processing as well. The earliest learned models for language generation typically consisted of material that was either a matter of public record or explicitly licensed for research use. All of that changed with the development of ImageNet, which now includes over 14 million hand-annotated images in its dataset. Created by AI researcher Fei-Fei Li (now at Stanford) and presented for the first time in 2009, ImageNet was one of the first cases of mass scraping of image datasets intended for computer vision research. According to Hanna, this qualitative scale shift also became the mode of operation for doing data collection, “setting the groundwork for a lot of the generative AI stuff that we’re seeing.” Eventually, datasets became so large that it became impossible to responsibly source and hand-curate datasets in the same way anymore. According to “The Devil is in the Training Data,” a July 2023 paper authored by Google DeepMind research scientists Katherine Lee and Daphne Ippolito, as well as A. Feder Cooper, a Ph.D. candidate in computer science at Cornell, “given the sheer amount of training data required to produce high-quality generative models, it’s impossible for a creator to thoroughly understand the nuances of every example in a training dataset.” Cooper, who, along with Lee presented a workshop at the recent International Conference on Machine Learning on Generative AI and the Law, said that best practices in training and testing models were taught in high school and college courses. “But the ability to execute that on these new huge datasets, we don’t have a good way to do that,” they told VentureBeat. By the end of 2022, OpenAI’s ChatGPT, as well as image generators like Stable Diffusion and Midjourney, had taken AI’s academic research into the commercial stratosphere. But this quest for commercial success — on a foundation of mass amounts of copyrighted data gathered without consent — hasn’t actually happened all at once, explained Yacine Jernite, who leads the ML and Society team at Hugging Face. “It’s been like a slow slip from something which was mostly academic for academics to something that’s strongly commercial,” he said. “There was no single moment where it was like, ‘this means we need to rethink everything that we’ve been doing for the last 20 years.’” But Databricks’ Rao maintains that we are, in fact, having that kind of moment right now — what he calls the “Napster moment” for generative AI. The 2001 A&M Records, Inc. v. Napster, Inc., landmark intellectual property case found that Napster could be held liable for infringement of copyright on its peer-to-peer music file sharing service. Napster, he explained, clearly demonstrated demand for streaming music — as generative AI is clearly demonstrating demand for text and image-generating tools. “But then [Napster] did get shut down until someone figured out the incentives, how to go back and remunerate the creators the right way,” he said. One difference, however, is that with Napster, artists were nervous about speaking out, recalled Neil Turkewitz, a copyright activist who previously served as an EVP at the Recording Industry Association of America (RIAA) during the Napster era. “The voices opposing Napster were record labels,” he explained. The current environment, he said, is completely different. “Artists have now seen the parallels to what happened with Napster – they know they’re sitting there on death’s doorstep and need to speak out, so you’ve had a huge outpouring from the artists community,” he said. Yet, industries are also speaking out — particularly in areas such as publishing and entertainment, said Marc Rotenberg, president and founder of the nonprofit Center for AI and Digital Policy, as well as an adjunct professor at Georgetown Law School. “Back when the Google books ruling was handed down, Google did very well in the outcome as a legal matter, but publishers and the news industry did not,” he said. The memory of that case, he said, weighs heavily. As today’s AI models require companies to hand over their data, he explained, a company like the New York Times recognizes that if their work can be replicated, they could go out of business (the New York Times updated its Terms of Service last month to prohibit its content from being used to train AI models). “To me, one of the most interesting legal cases today involving AI is not yet a legal case,” Rotenberg said. “It’s the looming battle between one of the most well regarded publishers, The New York Times, and one of the most impactful generative AI firms, OpenAI.” But lawyers defending Big Tech companies in today’s generative AI copyright cases say they have legal precedent on their side. One lawyer at a firm representing one of the top AI companies told VentureBeat that generative AI is an example of how every couple of decades a new, really significant question comes along and forms how the commercial world works. These legal cases, he said, will “play a huge role in shaping the pace and contours of innovation, and really our understanding of this amazing body of law that dates back to 1791.” The lawyer, who asked to remain anonymous because he was not authorized to speak about ongoing litigation, said that he is “quite confident that the position of the technology companies is the one that should and hopefully will prevail.” However, he emphasized that he thought those seeking to protect industries through these copyright lawsuits will have an uphill battle. “It’s just really bad for using the regulated labor market, or privacy considerations, or whatever it is — there are other bodies of law that deal with this concern,” he said. “And I think happily, courts have been sort of generally pretty faithful to that concept.” He also insisted that such an effort simply would not work. “The US isn’t the only country on Earth, and these tools are going to continue to exist,” he said. “There’s going to be a tremendous amount of jurisdictional arbitrage in terms of where these companies are based, in terms of the location from which the tools are launched.” The bottom line, he said, is “you couldn’t put this cat back in the bag.” Others disagree with that assessment: Rotenberg says the Federal Trade Commission is the one US agency with the authority and ability to act on these AI and copyright disputes. In March, the Center for AI and Digital Policy asked the FTC to block OpenAI from releasing new commercial versions of ChatGPT, citing concerns involving bias, disinformation and security. And in July, the FTC opened an investigation into OpenAI over whether the chatbot has harmed consumers through its collection of data. “If the FTC sides with us, they can require the deletion of data, the deletion of algorithms, the deletion of models that were created from data that was improperly obtained,” he said. And Databricks’ Rao insists that these generative AI models need to be — and can be — retrained. “I’ll be really honest, that even applies to models that we put out there. We’re using web-scraped data, just like everybody else, it has become sort of a standard,” he said. “I’m not saying that standard is correct. But I think there are ways to build models on permission data.” Hanna, however, pointed out that if there were a judicial ruling which found that generative AI could not be trained on copyrighted works, it would be “earth-shaking” — effectively meaning “all the models out there would have to be audited” to identify all the training data at issue. And doing that would be even harder than most people realize: In a new paper, “Talkin’ ‘Bout AI Generation: Copyright and the Generative AI Supply Chain,” A. Feder Cooper, Katherine Lee and Cornell Law’s James Grimmelman explained that the process of training and using a generative AI model is similar to a supply chain, with six stages — from the creation of the data and curation of the dataset to model training, model fine-tuning, application deployment and AI generation by users. Unfortunately, they explain, it is impossible to localize copyright concerns to a single link in the chain, so they “do not believe that it is currently possible to predict with certainty whether and when participants in the generative-AI supply chain will be held liable for copyright infringement.” The bottom line is that any effort to remove copyrighted works from training data would be incredibly difficult. Rotenberg compared it to asbestos, a very popular insulating material built into a lot of American homes in the 50s and 60s. When it was found to be carcinogenic and the US passed extensive laws to regulate its use, people had to take on the responsibility of removing it, which wasn’t easy. “Is generative AI asbestos for the digital economy?” he mused. “I guess the courts will have to decide.” While no one knows how US courts will rule in these matters related to generative AI and copyright, experts VentureBeat spoke to had varying hopes and predictions about what might be coming down the pike. “What I do wish would happen now is a more collaborative stance on this, instead of like, I’m going to fight it tooth and nail and fight it to the end,” said Rao. “If we say, ‘I do want to start permissioning data, I want to start paying creators in some ways to use that data,’ that’s more of a legitimate path forward.” What is causing particular angst, he added, is the increased emphasis on black box, closed models, so that people don’t know whether their data was taken or not and have no way of auditing. “I think it is actually really dangerous,” he said. “Let’s be more transparent about it.” Yacine Jernite agrees, saying that even some companies that had traditionally been more open — like Meta — are now being more careful about saying what their models were trained on. For example, Meta did not disclose what data was used to train its recently announced Llama 2 model. “I don’t think anyone wins with that,” he said. The reality, said lawyer Edward Klaris, is that the use of copyrighted works to train generative AI “doesn’t feel fair, because you’re taking everybody’s work and you’re producing works that potentially supplant it.” As a result, he believes courts will lean in favor of copyright owners and against technological advancement. “I think the courts will apply rules that did not apply in the Google books case, more on the infringement side,” he said. Karla Ortiz, a concept artist and illustrator based in San Francisco who has worked on blockbuster films including Marvel’s Guardians of the Galaxy Vol. 3, Loki, The Eternals, Black Panther, Avengers: Infinity War, and Doctor Strange, testified before the Senate hearing on AI and copyright on July 12 — so far, Ortiz is the only creative professional to have done so. In her testimony, Ortiz focused on fairness: “Ultimately, you as congress are faced with a question about what is fundamentally fair in American society,” she said. “Is it fair for technology companies to take work that is the product of a lifetime of devotion and labor, even utilize creators’ full names, without any permission, credit or compensation to the creator, in order to create a software that mimic’s their work? Is it fair for technology companies to directly compete with those creators who supplied the raw material from which their AI’s are built? Is it fair for these technology companies to reap billions of dollars from models that are powered by the work of these creators, while at the same time lessening or even destroying current and future economic and labor prospects of creators? I’d answer no to all of these questions.” The data underpinning generative AI has become a legal quagmire that may take years, if not decades, to wind its way through the courts. Experts agree that it is impossible to predict how the Supreme Court would rule, should a case related to generative AI and copyrighted training data come before the nine justices. But either way, it will have a significant impact. The unnamed Big Tech legal source VentureBeat spoke to said that he thinks “what we’re seeing right now is the next big wave of litigation over these tools that are going to, if you ask me, have a profound effect on society.” But perhaps the AI community needs to prepare for what they might consider a worst-case scenario. AI pioneer Andrew Ng, for one, already seems aware that both the lack of transparency into AI datasets, as well as the possibility of access to datasets filled with copyrighted material, could come to an end. “The AI community is entering an era in which we are called upon to be more transparent in our collection and use of data,” he admitted in the June 7 edition of his DeepLearning.ai newsletter The Batch. “We shouldn’t take resources like LAION for granted, because we may not always have permission to use them.”
Potential Supreme Court clash looms over copyright issues in generative AI training data
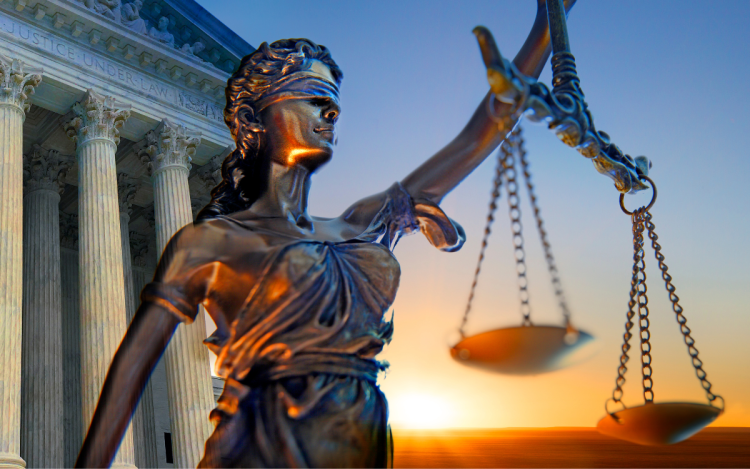